The Rise of Modern Data Governance
Data governance as a practice has had a rough journey – from being a helpful way to manage data, to becoming buried in red tape, and now emerging as a crucial enabler of innovation.
Data governance means different things to different people, making clear definitions essential. As I covered in Speaking Data Fluently: A Guide for Modern Data & AI Practitioners, understanding the terminology in your domain is critical, particularly for knowledge workers in technical fields like Data & AI.
The evolution and ambiguity around Data Governance, along with its fundamental importance today, is why I'm dedicating a comprehensive series to the topic.
This is Part 1 of The Datacraft Guide to Data Governance. In this installment, we'll understand how a practice meant to help teams work with data became synonymous with bureaucracy, and why it's now re-emerging as a critical foundation for innovation.
A Brief History: The Origins of Data Governance
The story of data governance begins with a simple but powerful premise: helping teams work better with data.
Before it acquired its corporate undertones, data governance emerged from data stewardship – where dedicated professionals acted as bridges between people and processes, bringing order to the chaos of early data management.
But you won't find much if you search for "the history of data governance." Why? Because somewhere along the way, data governance lost its appeal. It became synonymous with restrictions, regulations, and red tape – hardly the stuff of viral LinkedIn posts.
The 2008 financial crisis marked a turning point. As the mortgage market collapsed, regulators scrambled to prevent future disasters. This sparked the rise of compliance-focused governance frameworks and platforms like Collibra. Just like that, data governance became less about enabling teams and more about checking boxes and avoiding fines.
This shift created a persistent misconception. Today, many view data governance as the corporate equivalent of eating your vegetables – necessary but not particularly exciting. A task undertaken solely to avoid fines and security breaches. But this completely misses the original vision: data democratization and collaborative data management.
The irony? As organizations drown in more data than ever, we desperately need to return to those foundational principles.
True data governance isn't about control – it's about creating an environment where data can be trusted, found, and used effectively by everyone who needs it.
Data Governance Today: The Evolution
Data Governance has evolved beyond its compliance-focused roots to become the backbone of data strategy. Where organizations once viewed governance primarily through a compliance lens, it now encompasses a broader framework that ensures data remains valuable, accessible, useful, and credible - whether you're building AI models or analyzing market trends.
The numbers validate this evolution: Gartner reports 71% of organizations had Data Governance programs in 2024 — an 11% increase from 2023. The benefits are clear: 58% of organizations with data governance programs reported improved quality of data analytics and insights, as well as improved data quality.
But here's where things get interesting. Traditional data governance – you know, the kind with rigid top-down control and a lone data steward playing data police is becoming obsolete. In its place, a more flexible and inclusive approach is emerging.
Modern Data Governance is evolving in three fundamental ways: scope, ownership, and timing.
- From data governance to "data and analytics" governance: Think bigger. “Data” isn’t the only asset that needs to be governed anymore. Today's data assets include dashboards, machine learning models, code repositories, and more. Modern governance needs to handle it all.
- From a rigid centralized model to flexible governance models: The old model of centralized control through a lone data steward is evolving. Modern organizations typically adopt either a federated approach – where central teams set standards that business units implement locally – or a fully decentralized model where teams operate autonomously. Federated governance has emerged as a popular middle ground, offering the benefits of consistent standards while enabling teams to adapt practices to their specific needs. This evolution reflects today's workplace reality (especially Gen Z and millennials): teams want both clarity and autonomy in how they handle data. Top-down cultures are getting eroded and employees crave purpose in everything they do, so just telling people to do something won’t work anymore. Modern data governance is fundamentally practitioner-led. (Here's a short 2-min video to understand the differences between Centralized, Decentralized, and Federated)
- From an afterthought to a part of daily workflows: In the past decade, data governance was always applied as an afterthought. Data practitioners would ship projects as they were, then go back later and add data governance requirements dictated by top-down mandates. Forward-thinking organizations are baking governance into their daily workflows from day one instead of applying it retroactively.
Despite this evolution, organizations struggle to implement modern governance effectively. Gartner predicts that by 2027, 80% of data and analytics governance initiatives will fail due to a lack of a real or manufactured crisis.
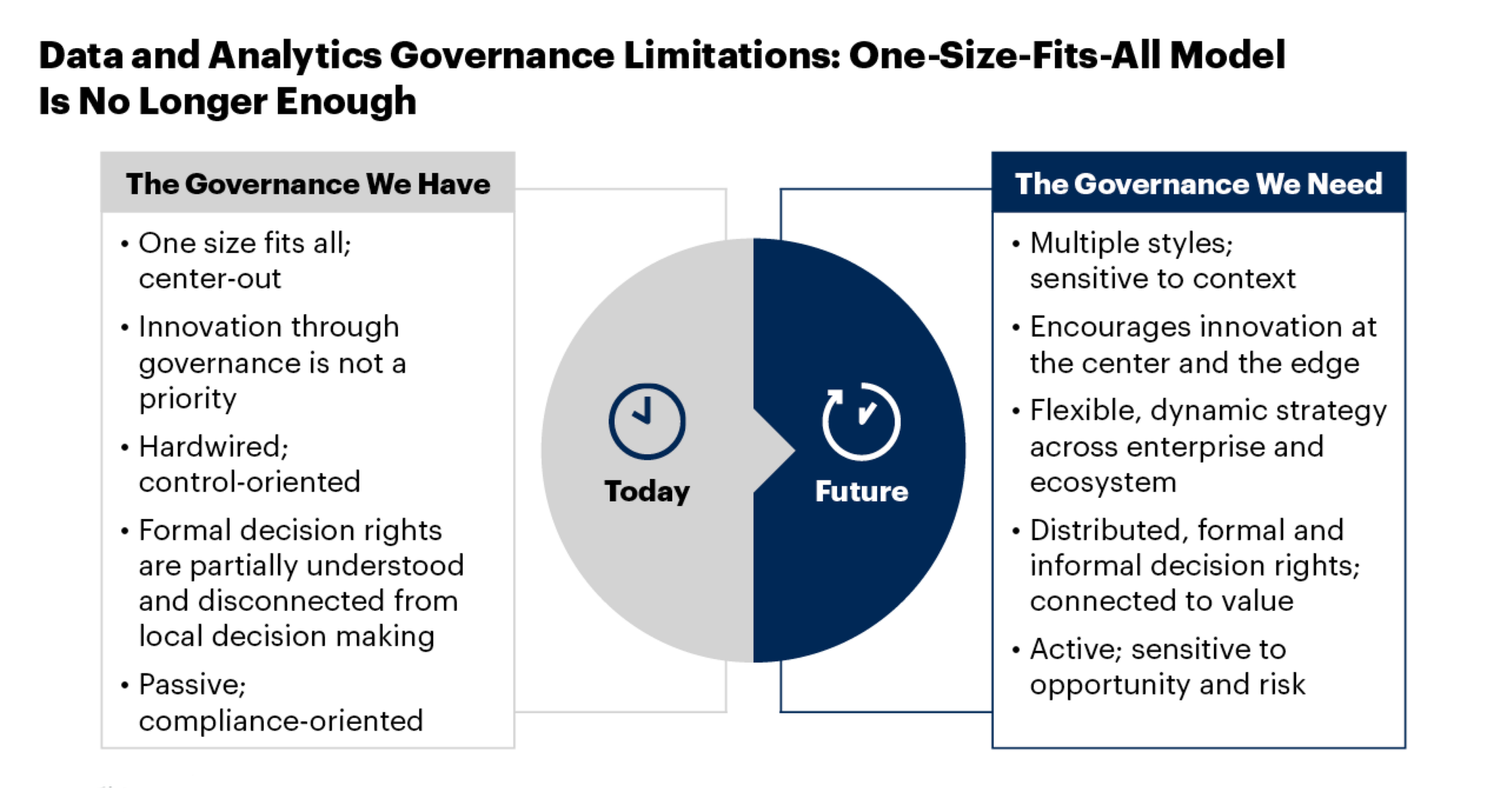
The good news? The global data governance market is expected to grow to $19.86 billion by 2032, reflecting substantial investment in this critical area. This underscores the need for organizations to approach data governance strategically and with a sense of urgency. Modern tools like Zeenea and Atlan are making governance less about bureaucracy and more about enablement. The transformation is real – data governance is becoming cool again.
Understanding the Basics of Data Governance
Before diving into specific aspects of data governance, let's understand it through a familiar analogy first. I firmly believe that if you can't explain something simply, you don't understand it well enough yourself.
Data Governance in Layman terms
Imagine moving into a new house with your family. You need systems for tracking documents, managing expenses, controlling access, and ensuring smooth operations. Some rules focus on security (who has spare keys), while others enable collaboration (shared calendars). That's essentially what data governance is for organizations – a system to manage and use data effectively.
What exactly is Data Governance?
Building on this analogy, we can define Data Governance as the internal framework that dictates how an organization manages, uses, and protects data assets through internal policies, standards, and controls to ensure compliance, data quality, and security.
It is fundamentally about creating order from chaos. Think of it like a constitution for your data: it provides the fundamental principles and rules that guide how your organization works with data.
The most important thing to understand about data governance is that it's not a technology solution or a one-time project - it's an ongoing discipline that requires commitment from across the organization.
Data governance relies on 3 interconnected elements: compliance as an essential outcome, data management operations to execute the rules, and data contracts to formalize how data should be exchanged and used.
Data governance and data compliance: So, they aren’t the same?
Data governance and compliance are terms often used interchangeably, but they serve fundamentally different purposes in your organization's data strategy. While compliance focuses on meeting specific regulatory requirements, governance encompasses a broader strategic framework that includes compliance as one of its key outcomes.
Think of data governance as your organization's internal playbook for managing data effectively, while data compliance is about meeting external rules set by regulators and industry standards. In fact, compliance requirements often represent just a subset of the controls and policies that a robust governance framework puts in place.
The main difference? Data governance is always proactive—you create internal frameworks and policies that dictate how your organization handles data. Data compliance requires proactive planning too, but because regulations continuously evolve and new ones emerge, organizations must remain responsive to changing external requirements. Even with established regulations like GDPR and HIPAA as a foundation, compliance practices need to adapt as interpretations change and new standards develop.
Returning to our household analogy helps clarify the relationship between governance and compliance. Your family's system for managing the household represents governance — it's your internal playbook for running things smoothly and achieving your goals. Compliance, by contrast, is like meeting building codes or homeowners' association rules. While these external requirements are important, they don't drive your fundamental decisions about how to organize and run your home. Similarly, effective Data Governance naturally enables compliance, but its primary purpose is creating value through better data management.
Here's the key: data compliance is actually an outcome of good data governance, not a separate process.
Data governance without compliance is ineffective; compliance without governance is impossible. They're two sides of the same coin, but governance is the side that determines the coin's value.
- Fenil Dedhia
Role of Data Compliance in Governance
Data compliance serves as a critical foundation within the broader governance framework. Here's how they work together:
- Governance Enables Compliance
- Creates clear accountability and ownership structures
- Establishes policies and procedures that embed compliance requirements into daily operations
- Implements controls and monitoring mechanisms
- Provides documentation and audit trails
- Compliance Strengthens Governance
- Provides clear requirements that inform governance policies
- Helps identify gaps in existing governance frameworks
- Drives continuous improvement in data handling practices
- Creates measurable standards for governance effectiveness
For a deeper dive into the relationship between governance and compliance, explore my dedicated article on Untangling Data Governance from Compliance.
Role of Data Management in Governance
While governance defines the rules, data management executes the day-to-day operations that handle data according to those rules. Think of it this way:
- Governance says "customer data must be encrypted at rest"
- Data Management handles the actual encryption, storage, and maintenance
Key management activities include:
- Data storage and archival operations
- Data processing and transformation
- Database maintenance and optimization
- Backup and recovery procedures
- Implementation of access controls
- Execution of data quality checks
Role of Data Contracts in Governance
Data contracts formalize the technical specifications for data exchange between providers and consumers. They serve as the detailed implementation guide for governance policies, specifically defining:
- Data structure and format requirements
- Quality standards and validation rules
- Access patterns and usage terms
- Service level expectations
- Integration specifications
For example, if governance policy requires "high-quality customer data," the data contract specifies exactly what that means:
- Required fields and their formats
- Acceptable values and ranges
- Quality metrics and thresholds
- Update frequency and freshness requirements
- Technical integration details
Think of data contracts as the bridge between governance principles and technical implementation. They translate high-level policies into specific, measurable, and implementable requirements that data management teams can execute.
Data Mesh: Rethinking Domain-Driven Data Governance
Domain-driven design has long guided how we structure software applications. Data Mesh extends these principles to data governance by recognizing a simple truth: the teams closest to business domains should govern their own data while adhering to organization-wide standards. Lack of domain know-how usually means that a centralized data management team will likely "mesh" it up ;).
A central team handling all data pipelines, quality, and access becomes a bottleneck, disconnecting domain experts from data ownership. Data Mesh solves this by distributing responsibility to domain teams who understand their data best.
This distributed approach naturally aligns with federated governance. Think of a modern retail chain: corporate headquarters provides standardized systems and tools (like point-of-sale and inventory management), while store managers make local decisions about inventory and customer service. Similarly in Data Mesh, a central platform team provides infrastructure and governance frameworks, while domain teams maintain autonomy over their specific data assets.
While this might sound similar to microservices architecture, the focus differs fundamentally. Microservices isolate application functionality with separate databases, but Data Mesh focuses on making domain data discoverable and usable across the organization. Where microservices manage transactional boundaries, Data Mesh enables cross-domain data sharing with clear ownership and governance standards.
The Data Mesh approach supports modern Data Governance through several key principles:
- Domain teams own both their operational processes and data quality
- Each domain provides data in standardized, consumable formats
- Governance standards apply consistently across domains while accommodating domain-specific needs
- Common infrastructure enables this distributed but connected approach
However, successful Data Mesh implementation requires careful evaluation. Organizations should assess whether their domain boundaries are well-defined and if their teams can handle expanded data quality and governance responsibilities. The goal isn't to distribute data management for its own sake, but to align data ownership with domain expertise while maintaining consistent governance standards.
Teams handling a large amount of data sources and a need to experiment with data (in other words, transform data at a rapid rate) would be wise to consider leveraging a data mesh. There are several calculators out there to determine if it makes sense for your organization to invest in a data mesh.
Legacy vs Modern Data Governance: 7 Key Differences
This evolution from traditional to modern Data Governance reflects broader shifts in how organizations work with data. Traditional approaches, born in an era of centralized data warehouses and strict regulatory responses, often created bottlenecks that hindered innovation. Modern governance recognizes that in today's data-driven world, governance must enable rather than restrict, while maintaining appropriate controls.
- Approach to Control
- Traditional: Centralized control with emphasis on hierarchical control over data access
- Modern: Federated or decentralized control, with emphasis on self-service and democratization
- Primary Goal
- Traditional: Compliance-first, treating governance as a necessary burden that often sacrifices innovation for control
- Modern: Value-first, viewing governance as a strategic enabler through data discovery and analytics
- Accountability
- Traditional: Overseen by select individuals or committees (Hierarchical org structure)
- Modern: Distributed responsibility - either through domain ownership with central guidance or fully autonomous domain teams
- Process Management
- Traditional: Manual processes requiring heavy documentation and approval chains
- Modern: Semi-automated or fully-automated workflows with embedded governance controls that are either shared or domain-specific
- Collaboration
- Traditional: Limited collaboration, typically within specialized data teams
- Modern: Cross-functional collaboration where teams actively participate in data decisions, encouraging input and shared accountability
- Technology Integration
- Traditional: Standalone governance tools disconnected from data platforms
- Modern: Governance capabilities integrated into daily workflows, either through shared platforms with domain customization or through domain-specific tooling
- Change Management
- Traditional: Reactive adaptation to new requirements, often resulting in outdated policies and missed opportunities
- Modern: Proactive evolution allowing domains to adapt quickly while maintaining organizational alignment through either shared frameworks or domain-specific approaches
The transformation from traditional to modern Data Governance mirrors the broader evolution in how organizations operate in the digital age. While traditional approaches emerged from an era of centralized control and regulatory compliance, they often created bottlenecks that stifled innovation and limited data value. Modern Data Governance recognizes a fundamental truth: in today's data-driven world, governance must act as an enabler of innovation while maintaining appropriate guardrails.
Success in modern Data Governance requires striking a delicate balance. Organizations must implement controls that ensure compliance and data quality without sacrificing the speed and flexibility that modern business demands. This balance isn't just about avoiding problems—it's about creating competitive advantage.
Member discussion