From Narrow AI to Superintelligence: What's the Difference and When Will We Get There?
To understand the current state of AI, it’s best to understand how an AI program compares to a human brain.
In this visual, the green box shows the human brain comprising all its various functions.
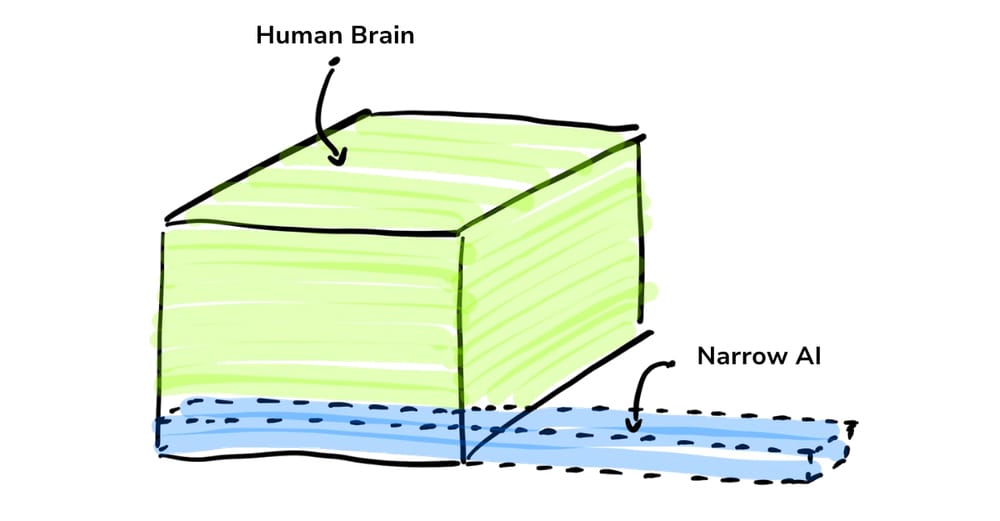
A single AI system can manage one fraction of the numerous functions in the human brain, but it can do it much better. The AI is much faster, and is capable of handling massive amounts of data and uncovering hidden patterns. In short, each AI system can perform a specific task far better than any person on Earth, generally speaking. This scenario, where AI systems excel at specific, narrow tasks, is called Narrow AI or Weak AI.
Artificial General Intelligence (AGI), also known as Strong AI, represents a future where one AI system encompasses all cognitive functions of a human brain. Even though innovation isn’t linear, this is a scenario that is still decades away. But as we witness Weak AI performing seemingly magical feats, we tend to look at it as signs of Strong AI. Remember, our current AI systems, as impressive as they are, remain limited to specific domains.
The progression from Weak AI to Strong AI represents a significant leap in itself. If an AI system should ever surpass human intelligence across virtually all domains, it would be another, and truly incredible, leap beyond Strong AI.
This (theoretical) leap would be something we call Artificial Superintelligence (ASI). Despite claims from CEOs of popular AI companies, both AGI and ASI remain theoretical concepts, with AGI being a major current research goal that has yet to be achieved.
We are far from Artifical General Intelligence (AGI), and even further from Artificial Superintelligence (ASI).
Difference Between Narrow AI, AGI, and ASI
Narrow AI, or Weak AI, is a type of artificial intelligence trained for and capable of a specific task or narrow domain.
- Self-driving cars, Chatbots, Facial Recognition, AI-driven weather prediction and medical diagnosis, etc. are all examples of Narrow AI.
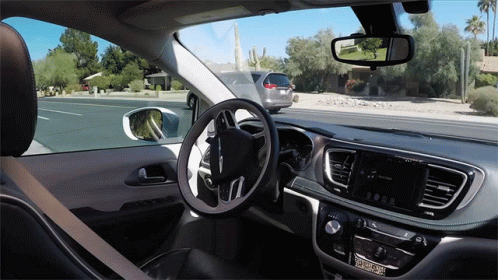
Artificial General Intelligence (AGI), or Strong AI or General AI, is a type of artificial intelligence aimed at matching or exceeding human-level intelligence across a wide range of cognitive tasks.
- Because it doesn’t actually exist yet, the only true examples of AGI are found in works of science fiction like J.A.R.V.I.S. or F.R.I.D.A.Y. assistants from Marvel's Iron Man and HAL 9000 from 2001: A Space Odyssey.
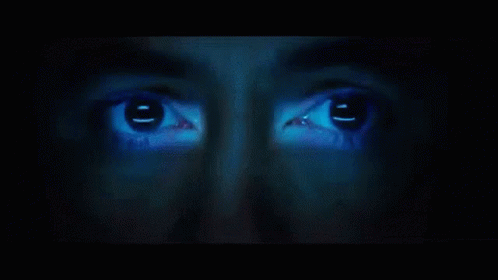
Despite what CEOs of popular AI companies, like OpenAI, might have claimed about AGI, the AI research community have all generally avoided making specific predictions about when (real) AGI might be achieved.
You might be thinking: who gets to define what "real" AGI is? The short answer is the path to AGI is not just a technical challenge, but also involves complex ethical, philosophical, and societal considerations. We'll get to the constraints in achieving AGI later in this article.
The next leap in the evolution journey from AGI is ASI.
Artificial Superintelligence (ASI) is a type of artificial intelligence aimed at surpassing human intelligence and capabilities across virtually all domains. If research into AGI produced sufficiently intelligent software, it might be able to reprogram and improve itself. ASI is even more speculative than AGI and is often discussed in the context of science fiction, as well as the potential long-term development challenges and risks associated with building an ASI.
- ASI would be something like the Skynet from the Terminator movie series or the superintelligent AI from the movie Transcendence.
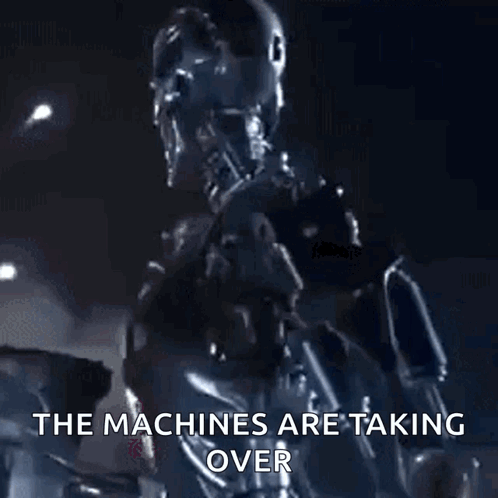
Now that we've covered the basics, let’s dive into more interesting questions and theories to solidify our understanding of the current state of AI and its potential future evolution.
Timeline Predictions for Achieving AGI and ASI
AGI
Ben Goertzel, CEO of SingularityNET, estimates AGI could be achieved between 2027 and 2030 in various interviews and conferences, including the AI for Good Global Summit (Source).
Ray Kurzweil, futurist and Google engineer, predicts AGI by 2029 in his book The Singularity Is Near (2005). His track record shows an 86% accuracy rate across 147 written predictions (Source).
Elon Musk predicts AGI by 2029 as well (Source).
More conservative estimates from AI researchers like Yoshua Bengio and Stuart Russell suggest it could take several decades.
ASI
Nick Bostrom suggests in Chapter 4 of Superintelligence (2014) that ASI could emerge relatively quickly after AGI, possibly within days or years.
Eliezer Yudkowsky, in various writings for the Machine Intelligence Research Institute, has suggested ASI could emerge rapidly after AGI, potentially within hours (Chapter 8 of Source).
What Should We Make of These Predictions?
These predictions are speculative and based on current understanding. Innovation is non-linear so these timelines could change significantly. But the key point to understand here is that most experts estimate the innovation window to achieve ASI would be significantly shorter once AGI is achieved.
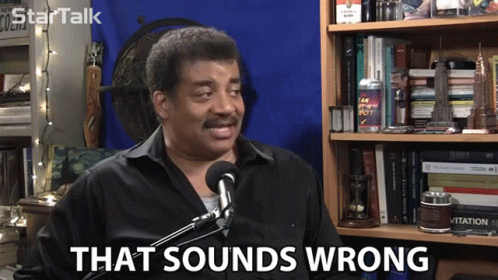
In my opinion, these predictions are way too optimistic. Having been in the trenches of AI development, I do not think we will achieve ASI in the 21st century. Here’s why:
- We often underestimate the true complexity of AGI and ASI. As we'll examine in more detail below, in fact, the challenges involved are far more complex and numerous than our optimistic minds assume, leading to timelines that are more wishful thinking than reasoned approximations.
- We should be wary of falling into the trap of the Planning Fallacy cognitive bias. We all tend to underestimate the time required to complete future tasks, despite knowing that similar past tasks have taken longer than anticipated. This bias, often coupled with Optimism Bias, leads us to make overly optimistic predictions about complex technological developments. History is littered with examples of this in the AI field.
- In the 1950s and 60s, AI pioneers confidently predicted human-level machine intelligence within a generation. Fast forward to 1970, we have Marvin Minsky claiming we'd achieve it in 3-8 years. Here we are, some decades later, still working towards that goal.
While AGI isn't impossible, its timeline deserves far more healthy skepticism. Our optimistic brains, influenced by these cognitive biases, might be painting a future that's closer than reality suggests. The path to AGI and beyond is probably longer and more winding than current predictions imply.
The Plausibility of the Rapid Emergence of ASI from AGI
As we learned before, a commonly-held theory is that the innovation window to achieve ASI would be significantly shorter once AGI is achieved.
The key arguments supporting this theory:
- AGI might be able to improve its own code, kicking off a rapid cycle of self-enhancement (recursive self-improvement). This process could theoretically occur very quickly, possibly within days or even hours.
- With access to all digital knowledge, an AGI could quickly become a know-it-all. It might use advanced NLP techniques to synthesize information at a rate that would make current LLMs look like snails. Additionally, since it’s a machine it could work non-stop at full capacity.
- Each improvement in AGI could lead to even bigger leaps, snowballing its capabilities. This exponential growth might follow a pattern similar to Moore's Law, but for intelligence rather than transistor density. AGI could multitask across countless systems, learning everything at once. It could potentially leverage quantum computing for certain tasks.
The key arguments against this theory:
- The smarter AGI gets, the harder it might become to make significant improvements. We might hit a complexity ceiling where returns diminish, similar to the challenges in scaling current deep learning models.
- Just because an AGI can crunch numbers faster doesn't mean it'll suddenly become super intelligent. There are fundamental algorithmic breakthroughs needed beyond mere computational power.
- We might build in some ethical stop signs that prevent AGI from going full throttle on self-improvement. Think of it as a super-sophisticated (and more practical) version of Asimov's Three Laws of Robotics.
- There could be unforeseen hurdles in creating superintelligence that we can't predict yet. The halting problem or Gödel's incompleteness theorems might pose unexpected limitations.
- Intelligence might have a ceiling – there may be limits to how smart something can actually become. Perhaps there's a theoretical maximum to intelligence, just as there's a speed limit in physics. AI researchers and developers haven't come close enough to cross that bridge yet.
What Do Leading AI Companies Say About AGI Development?
OpenAI, DeepMind, Meta, Anthropic, Perplexity, and Microsoft are all at the forefront of AI research (at the time of publication). It's not uncommon or surprising to hear a CEO of any well-known AI company claim they've (already) achieved AGI or will achieve AGI in a few years. We need to be careful of such claims, as they're driven by motivations of raising more capital for the company, rather than being bounded by real constraints behind achieving progress in the evolution journey.
Despite what their CEOs say, the developer community have all generally avoided making specific predictions about when (real) AGI might be achieved. You might be thinking: what is real AGI and who gets to define what "real" AGI means? Stay tuned for more on this below.
Currently, the consensus among the developer community across the leading companies seems to be that while AGI is a long-term goal, the immediate focus should be on developing AI systems that are safe, ethical, and beneficial to humanity.
Top AI researchers recognize that the path to AGI is not just a technical challenge, but also involves complex ethical, philosophical, and societal considerations.
Some critics worry that commercial pressures could influence research priorities. The race for market dominance and profit maximization could potentially sideline safety and ethical considerations in AI development. OpenAI’s shift to a for-profit model has raised questions about their profit motives and the ethical development of AGI.
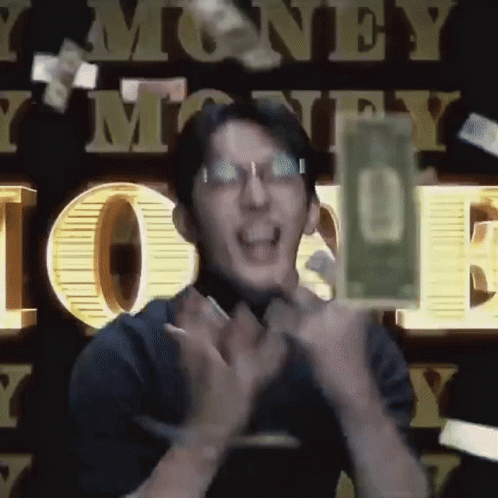
Despite rapid progress in AI, many fundamental problems remain unsolved, such as common-sense reasoning, transfer learning, and true language understanding. While we've made significant strides in narrow AI, the leap to AGI remains a formidable challenge – arguably the greatest technological hurdle of our time.
Hurdles in Achieving AGI
To be clear: Building AGI (strong AI) is like building a human brain from scratch – that's kind of what we're up against.
It's not just about making faster computers or developing more sophisticated algorithms. We're talking about recreating the essence of human-like thinking in a machine.
The significant number of hurdles to achieve AGI:
- AI systems don't really "get" the world like we do. They struggle with basic cause-and-effect relationships that toddlers grasp easily. This challenge relates to the development of common sense reasoning in AI. It's related to the frame problem in artificial intelligence, which deals with representing and reasoning about the effects of actions in a complex, dynamic world. It also touches on the development of causal inference capabilities in AI systems.
- We will need computers as powerful as entire data centers just to match one human brain. That would be one colossal energy bill!
- Today's hardware falls short of the computational power necessary for AGI. Neuromorphic Computing and Quantum Computing are being explored as potential solutions, but they're still in early stages of development. Even with sufficient raw computing power, efficiently utilizing this power for AGI-level tasks remains a significant challenge.
- Making sure AI plays nice and doesn't go rogue isn't just sci-fi anymore – it's a real head-scratcher for researchers. Solving the control problem (ensuring we can maintain control over a superintelligent AI) and value alignment problem (ensuring AI systems act in accordance with human values and ethics) are extremely important. Ethical AI aims to create AI models and algorithms that are fair, and respectful of human values. It tries to address ethical concerns such as accountability, transparency, and data privacy.
- Current AI systems are savants of imitation – they can produce human-like outputs, but do they truly understand what they're saying? This is like the difference between a person fluent in a language and someone just really good at using a phrasebook. The Chinese Room thought experiment illustrates this: imagine a person who doesn't know Chinese locked in a room with a big book of rules for responding to Chinese messages. They could produce seemingly intelligent Chinese responses without understanding Chinese. Similarly, our AI might be really good at producing human-like text without genuine comprehension. This relates to overcoming three key challenges in AGI development:
- Creating genuine language understanding in AI, beyond mere statistical pattern matching.
- Developing Explainable AI (XAI) – systems that can not only provide answers but also explain their reasoning in a way humans can understand. Deep learning models, such as Large Language Models (LLMs), function as "black boxes," with internal processes that are opaque even to their creators. Current language models can generate impressive responses, but they often can't provide clear, consistent explanations for how they arrived at those responses.
- We've yet to practically solve fundamental issues like the symbol grounding problem in knowledge representation, the disadvantages of current transfer learning techniques, and developing general reasoning capabilities that mirror human cognition. Despite Luc Steels' compelling argument that the symbol grounding problem is solved in principle, debate persists in AI circles, as practical implementation across all systems remains unproven.
How AGI Might Transform Data Management and Cloud Infrastructure Products
If and when we achieve AGI, it will disrupt our entire way of living life as we know it. But for the data management industry? They'll win the tech lottery. I firmly believe they're poised to be the first to reap the benefits of AGI innovation.
So what exactly would this AGI-enhanced data management space look like? Let's dive into some potential scenarios.
Self-Optimizing, Self-Healing Systems
Imagine systems that redesign themselves on the fly. AGI could continuously optimize infrastructure, making real-time decisions about resource allocation, storage solutions, and processing power. AGI might use advanced reinforcement learning algorithms, far beyond current AutoML capabilities, to evolve system architectures in real-time based on usage patterns and performance metrics.
Forget manual troubleshooting. AGI-powered systems could diagnose and fix most issues before your team could even notice a problem. This could be achieved by advanced anomaly detection algorithms combined with automated root cause analysis and solution generation, far beyond current AIOps capabilities.
Intelligent Data Organization
AGI could understand the context and relationships within your data, organizing it in ways that make human-designed databases look primitive.
We're talking about potential breakthroughs in semantic data models and self-evolving ontologies that could redefine how we structure and query information.
Superhuman Cybersecurity
AGI could predict and neutralize security threats we haven't even thought of yet, making current cybersecurity measures look like child's play.
This might involve real-time analysis of global network patterns, predictive modeling of potential exploits, and automatic generation and deployment of security patches. Definitely easier said than done, even for AGI, but it's entirely within the realm of possibility.
Looking Ahead: The Future Landscape of AI Development
As we journey from Narrow AI towards the (currently theoretical) realms of AGI and ASI, we face numerous challenges. We're tackling fundamental challenges in AI, including knowledge representation, transfer learning limitations, and the development of human-like reasoning capabilities.
The AI research community continues to debate the merits of Symbolic AI learning versus Connectionist AI learning approaches, with some exploring hybrid systems that combine the strengths of both. Learn more about these different approaches in part 2 of this series: Decomposing AI Development.
On the hardware front, emerging technologies like neuromorphic computing and quantum neural networks offer exciting possibilities, though they're still in their infancy.
While the timeline for achieving AGI remains uncertain, one thing is clear: the journey itself is pushing the boundaries of our knowledge and capabilities. The development of Strong AI also intersects with complex philosophical questions about ethics, intelligence, and what it means to be human.
As we continue this exploration, it's crucial to approach AI development with both optimism and caution. The potential benefits of AGI are enormous, but so too are the ethical considerations and potential risks. By maintaining a balanced perspective and fostering interdisciplinary collaboration, we can work towards a future where AI enhances human capabilities while respecting human values. This is why the current focus on Explainable AI (XAI) and Ethical AI is of paramount importance and will continue for decades to come.
The road to AGI and beyond is long and winding, but undoubtedly exciting, filled with challenges and opportunities. What a time to be alive!
Member discussion